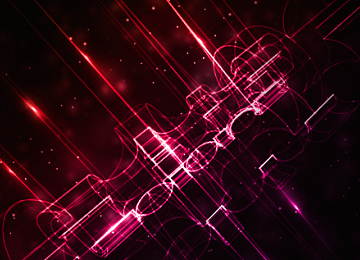
Blog
Reflection
from
A CFW
Artist-in-
Residence
By: Jane Adams
In its original conception, the piece was intended to expose people to the collective behavior that they participate in while going about their individual day-to-day business. The first challenge was thus identifying a dataset that was both individually and collectively interesting; the second was to display that dataset in a way that was both individually and collectively interesting. While the MTA turnstile dataset does, I believe, live up to the first challenge, the piece as it stands today does not do as much for the second challenge as it could.
My intentions shifted away from exposing collective behavior toward exposing the realities of data that is, without question, about each of us. While there seems to be a growing awareness that there is data about us, there doesn’t seem to be a growing awareness of what exactly the upshot of that is. I have heard many rail against the collection (and usually resale) of data about them, but I’ve heard far fewer detailing why the collection and use of data about individuals, especially without their consent, awareness, and understanding, is problematic.
Data feels abstract, disembodied. It doesn’t feel like it’s about us. There are surely those that benefit from this persisting as the broad cultural attitude toward data about the participants in that culture. While working on the piece I became more and more aware of the need to contextualize, and humanize, what it means for there to exist data about us. It is crucial for individuals to understand 1) what “data about us” captures and, importantly, doesn’t capture, and 2) what it is used for and how. The intersection of these two is where we begin to develop and advocate for better and fairer data practices.
For example, it’s reasonable to assume that the MTA uses, among other datasets, the turnstile data to make decisions about line repairs and station closures, to identify and prioritize the repair of vulnerabilities, etc. Here the quality of the data is paramount. While working on the piece I noticed that the naming of stations within the dataset is not consistent (this is just one of the data quality issues in this dataset). “How might inconsistent station-naming impact decision-making that will affect my day-to- day?” This is what I now hope someone will ask when engaging with this piece. If, say, for a few months the subway station in your neighborhood that you use every day is spelled slightly differently, how is that being accounted for? Are those records with the misspelled station name left out? Are station names “fuzzily” matched in a way that might accidentally include stations that aren’t the right station? How does the MTA use turnstile data in its decision-making in the first place? Drawing from real-world examples such as hurricanes and presidential visits, I want to simulate what the decision-making process using different approaches to the data itself to reveal how far outcomes can diverge from one another due to seemingly superficial decisions about the data driving them.
In this newer conception, it will be important to find a way to embed the piece in historical contexts of both mass transit and data collection. This is probably what I’m most enthusiastic about. It will also be important to make the data quality issues in this dataset more obvious, more accessible. Much of data work proceeds by smoothing over and hiding such issues. The challenge is that, often, data quality issues are visually jarring. The stream graph form the piece takes today is itself a departure from its original form (a map); there may yet be another form in the piece’s future.
In summary, I feel that the residency afforded me the time, space, and resources to engage with data in a way that is largely new to me. As a practitioner, the outcome of that engagement feels relevant and important beyond my own edification. My thinking about data literacy (it may not be obvious, but much of the above is ultimately about data literacy) went through something of a growth spurt during the residency: nascent feelings about data’s importance in everyday life were given the opportunity to surface and mature; vague frustrations about data misuse and data-driven abuse became tools instead of hindrances. I am excited to keep engaging.